Data quality has become increasingly important in recent years, particularly in the automotive industry. This data is used to inform everything from vehicle design to maintenance and repair, making data quality a critical factor in the success of automotive businesses. In this blog, we’ll explore how data quality impacts automotive profiles, and why it’s important for businesses to prioritize data quality in their operations.
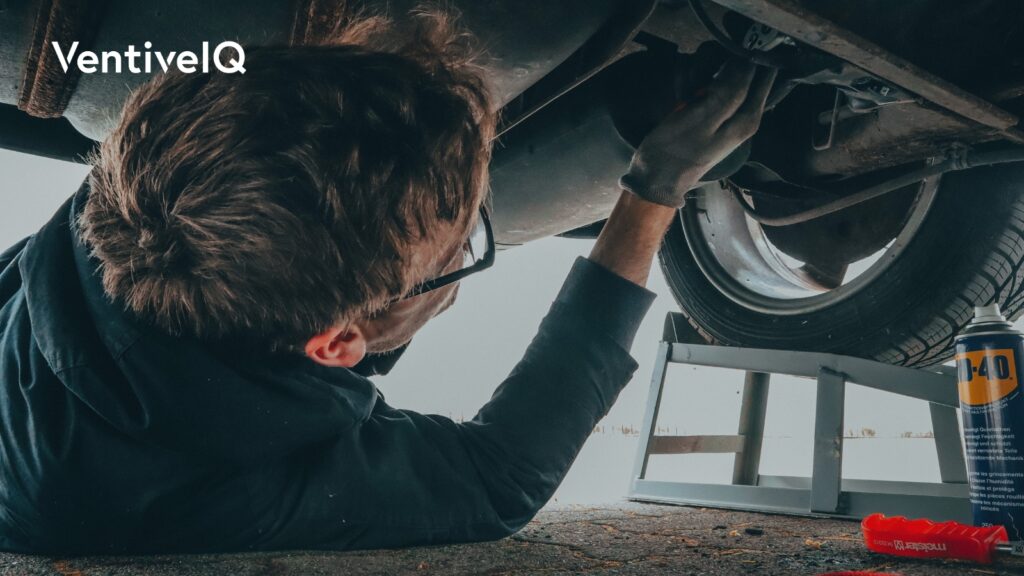
First, let’s define what we mean by “Automotive Profiles.” In the context of this blog, we’re talking about the data that is collected and analyzed by automotive businesses to understand customer behavior, vehicle performance, and other important factors. This includes everything from vehicle sensor data to customer purchase history and demographic information. By analyzing this data, businesses can make informed decisions about everything from product design to marketing campaigns.
So, how does data quality impact automotive profiles? The answer is in many ways. Poor data quality can lead to incorrect conclusions, missed opportunities, and increased costs. For example, if a business is using inaccurate customer data to inform its marketing campaigns, it may end up targeting the wrong audience or missing potential customers altogether. Similarly, if a business is using faulty sensor data to inform its vehicle design, it may end up creating a product that performs poorly or has safety issues.
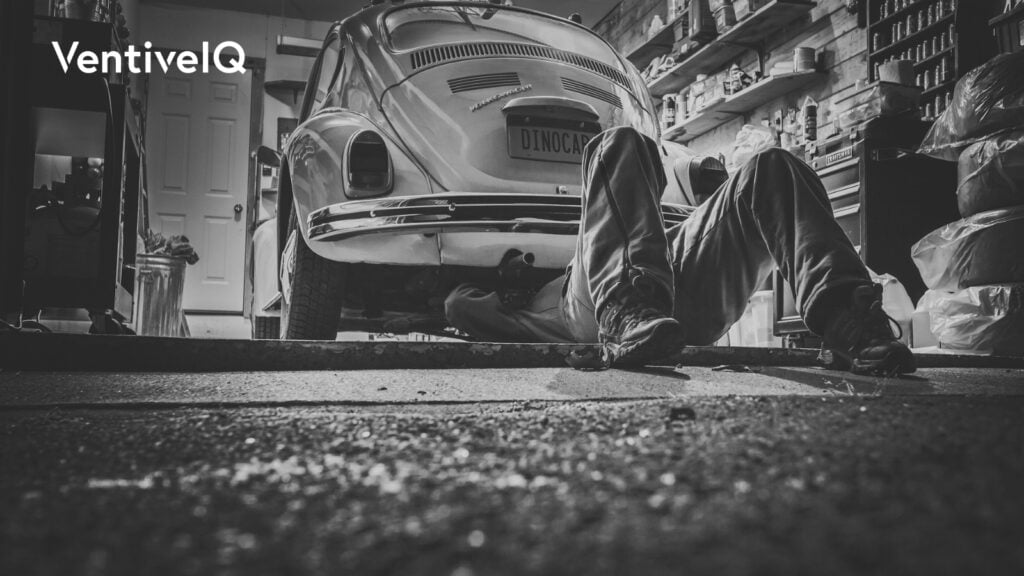
One of the most significant impacts of poor data quality on automotive profiles is the potential for increased costs. When data is inaccurate, it can lead to incorrect decisions, which can result in wasted time, resources, and money. For example, if a business is using faulty data to inform its inventory management system, it may end up ordering too much or too little of certain products, which can lead to excess inventory or stock shortages. These issues can be costly, both in terms of lost revenue and increased expenses.
In addition to increased costs, poor data quality can lead to missed opportunities. When businesses are unable to accurately analyze data, they may miss out on opportunities to improve their operations or gain a competitive advantage. For example, if a business is not collecting or analyzing customer feedback data, it may miss out on opportunities to improve its product offerings or customer service. Similarly, if a business is not accurately analyzing vehicle sensor data, it may miss out on opportunities to identify and address performance issues.
On the other hand, high-quality data can lead to a variety of benefits for automotive businesses. Accurate and reliable data can inform better decision-making, leading to improved operations, increased efficiency, and better customer experiences. For example, if a business is accurately collecting and analyzing customer feedback data, it may be able to identify areas for improvement in its product offerings or customer service, leading to increased customer satisfaction and loyalty. Similarly, if a business is accurately analyzing vehicle sensor data, it may be able to identify and address performance issues before they become major problems, improving the safety and reliability of its products.
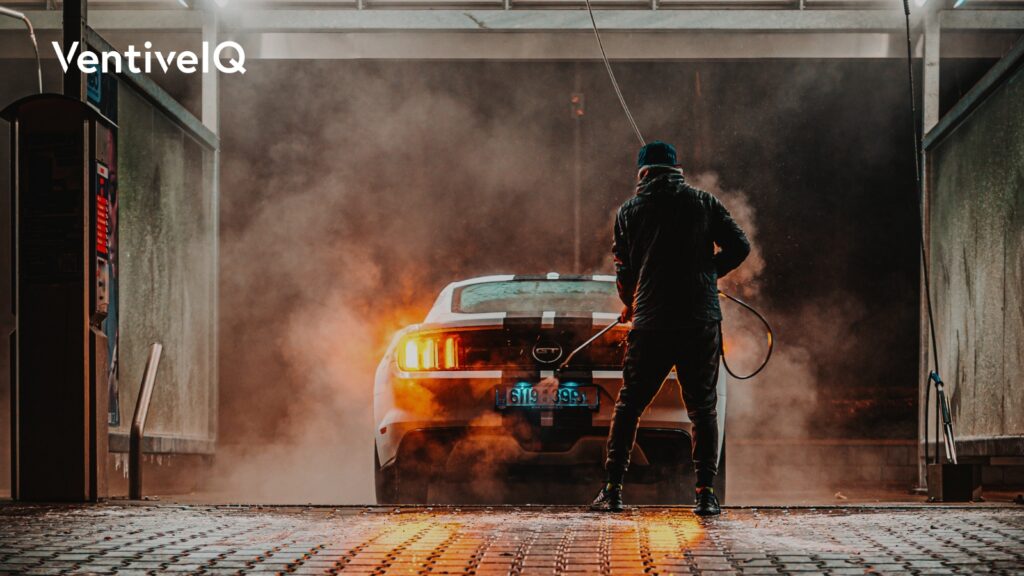
So, how can automotive businesses ensure high-quality data? There are several strategies that businesses can employ to improve their data quality, including:
- Data validation and cleansing: As mentioned earlier, data validation and cleansing is an essential step in ensuring high-quality data. Businesses should employ automated tools and processes to help identify and correct errors, inconsistencies, and inaccuracies in the data. This can include tools like data quality software or machine learning algorithms.
- Standardization: Standardizing data can help ensure that it is consistent and accurate across different systems and processes. Businesses should establish consistent data formats, naming conventions, and other standards that all data must adhere to. This can help reduce errors and inconsistencies in the data and make it easier to analyze and interpret.
- Data governance: Establishing strong data governance practices can help ensure data is managed effectively and securely throughout its lifecycle. This includes defining roles and responsibilities for data management, establishing data quality standards, and implementing processes for data storage, backup, and recovery.
- Data integration: Integrating data from different sources can help provide a more complete picture of customer behavior, vehicle performance, and other important factors. Businesses should ensure that they are using tools and processes that enable seamless data integration across different systems and platforms.
- Data quality monitoring: Businesses should regularly monitor their data quality to ensure that it meets their standards and expectations. This can include using tools like data quality dashboards or automated alerts to identify data quality issues and take corrective action.
- Data training: Ensuring that employees are trained in data quality best practices is essential to improving data quality. Businesses should invest in training programs that help employees understand the importance of data quality, how to identify and correct data quality issues, and how to use data effectively to inform decision-making.
VentiveIQ has almost 200 million verified vehicle owner records from secure and privacy-compliant auto data sources. Each record is linked with a Persistent ID, Household ID, and demographic data for accurate targeting. The auto owner profiles are compiled from various sources, including sales and service data, warranty information, and maintenance records.
Conclusion
Improving data quality requires a concerted effort from businesses, including investment in the right tools, processes, and training programs. By prioritizing data quality, businesses can improve their operations, reduce costs, and gain a competitive advantage in the automotive industry. Data quality is critical for automotive profiles, impacting every aspect of the automotive value chain. By investing in data quality tools and technologies and developing data governance policies and procedures, automotive companies can ensure that their data is accurate, complete, and timely, leading to better decision-making, improved customer service, and increased revenue.