Predictive analytics is a powerful tool that can help businesses make informed decisions based on historical data and future trends. By analyzing large amounts of data and using machine learning algorithms, businesses can make predictions about future events, customer behavior, and market trends. In this blog, we’ll explore the top 5 real-world examples of predictive analytics in action and how brands are leveraging data to drive results.
Top 5 Real-World Examples of Predictive Analytics
Predictive analytics is a branch of data analytics that uses statistical algorithms and machine learning techniques to analyze historical data and make predictions about future events or trends. Here are some top 5 real-world examples of predictive analytics in action.
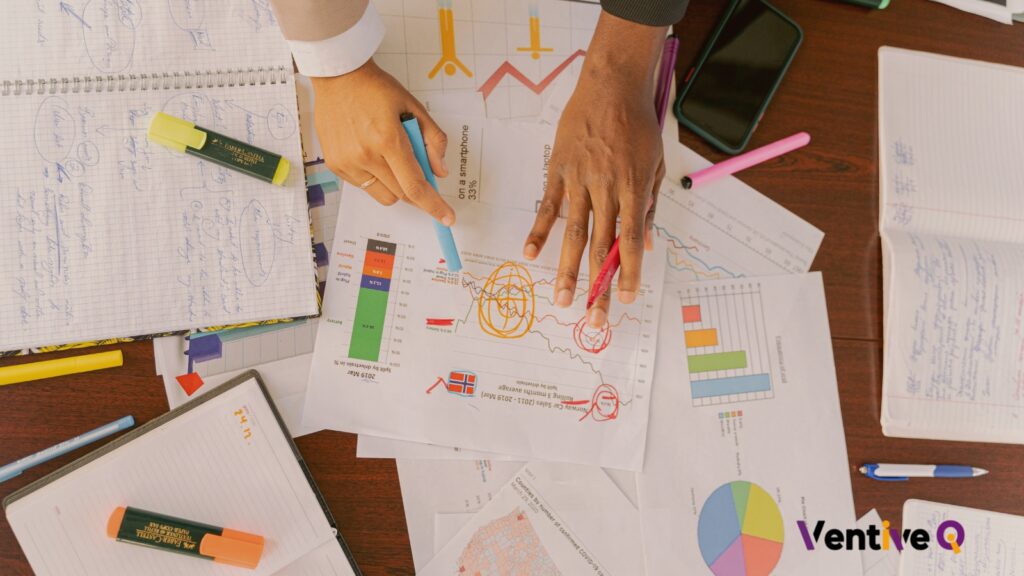
Know more about Data Segmentation and its significance.
1. Retail: Amazon
Amazon is a leader in predictive analytics. The company uses machine learning algorithms to analyze customer data and make personalized product recommendations. Amazon’s recommendation engine analyzes customer data such as purchase history, browsing behavior, and demographic information to suggest products that the customer is most likely to purchase. This approach has been incredibly successful for Amazon, with the company reporting that personalized recommendations account for up to 35% of its revenue.
2. Finance: American Express
American Express uses predictive analytics to identify customers who are at risk of canceling their credit cards. The company analyzes data such as payment history, credit scores, and spending patterns to identify customers who are most likely to cancel their cards. American Express then reaches out to these customers with targeted offers and incentives to encourage them to keep their cards. This approach has been successful, with the company reporting that it has been able to reduce card cancellations by 400%.
3. Healthcare: Sutter Health
Sutter Health, a healthcare provider based in California, uses predictive analytics to improve patient outcomes and reduce costs. The company uses machine learning algorithms to analyze patient data such as medical history, lab results, and medications to identify patients who are at risk of readmission or complications. Sutter Health then intervenes with targeted care plans and interventions to help these patients stay healthy and avoid costly readmissions. This approach has been successful, with the company reporting that it has been able to reduce readmissions by 27%.
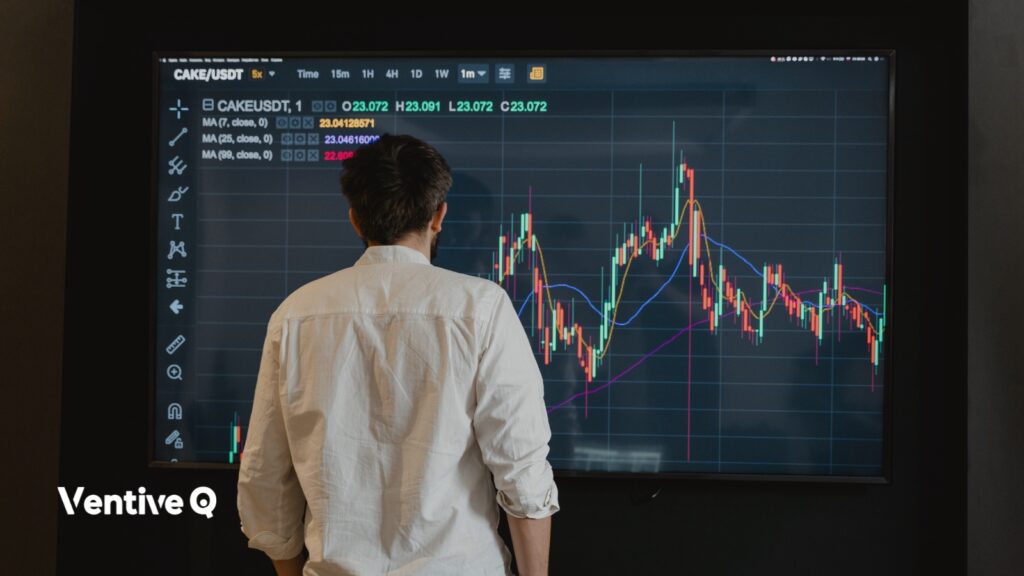
4. Manufacturing: Caterpillar
Caterpillar, a heavy equipment manufacturer, uses predictive analytics to improve maintenance and reduce downtime. The company uses sensors and machine learning algorithms to analyze data such as equipment usage, operating conditions, and maintenance history to predict when equipment is likely to fail. Caterpillar then schedules maintenance before the equipment fails, reducing downtime and improving overall productivity. This approach has been successful, with the company reporting that it has been able to reduce downtime by up to 55%.
Learn more about personalized marketing and it drives engagement and loyalty.
5. Transportation: UPS
The utilization of predictive analytics by UPS enables it to enhance its delivery routes and minimize fuel consumption. The company uses machine learning algorithms to analyze data such as traffic patterns, delivery volume, and driver behavior to optimize delivery routes in real time. This approach has been successful, with the company reporting that it has been able to reduce fuel consumption by 10 million gallons per year.
The real-world examples mentioned above show how predictive analytics can be used across a wide range of industries to drive business outcomes.
How Brands Are Leveraging Data to Drive Results
In today’s digital age, data is everywhere. From social media metrics to website analytics, companies have access to a wealth of information about their customers and their behaviors. This data is invaluable when it comes to driving business results and improving the overall customer experience. As a result, brands are increasingly leveraging data to make more informed decisions and drive success.
One way in which brands are leveraging data is through customer relationship management (CRM) systems. CRM software allows companies to collect and analyze customer data in order to better understand their needs, preferences, and behaviors. By using this information, brands can create more targeted and personalized marketing campaigns, improve customer service, and drive sales.
Discover Creative Examples of Personalized Marketing Campaigns That Leveraged Customer Data!
For example, a clothing retailer may use a CRM system to track customer purchases and preferences. They may use this data to create targeted email campaigns that promote specific products or styles based on a customer’s past purchases. This approach can be highly effective, as customers are more likely to respond to messages that are tailored to their individual needs and interests.
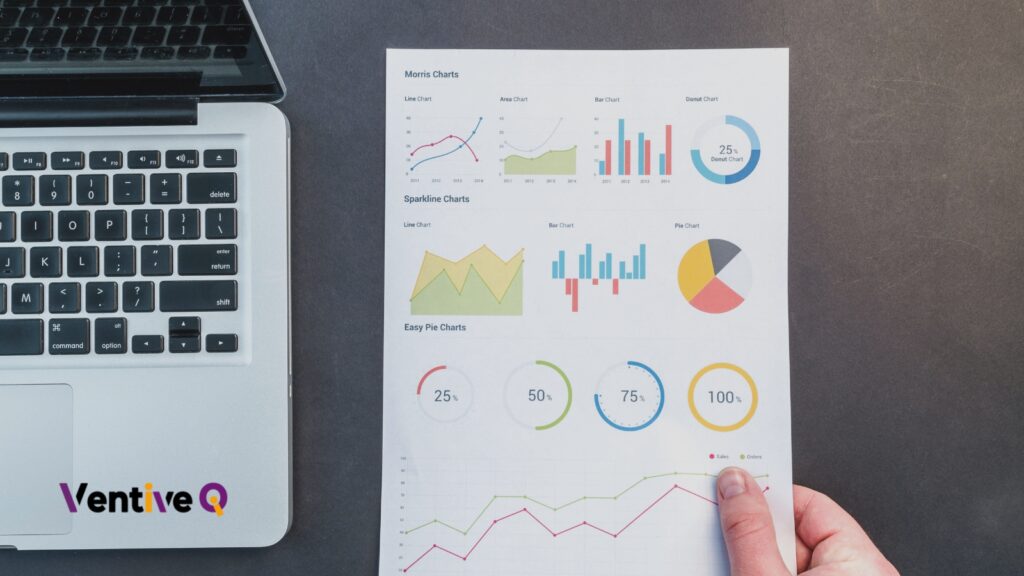
Another way in which brands are leveraging data is through the use of social media analytics. Social media platforms provide a wealth of information about customer behavior, including likes, comments, and shares. Brands can use this data to gain insights into customer preferences and opinions and to create more engaging content that resonates with their audience.
In addition to CRM systems and social media analytics, brands are also leveraging data through the use of machine learning and artificial intelligence (AI). These technologies allow companies to analyze vast amounts of data and make predictions about customer behavior.
By utilizing VentiveIQ’s CPG data, you can effectively target shoppers during their purchasing decision-making process, while simultaneously reducing your time, energy, and financial investment. Discover the audience that views your advertisements and the manner in which they perceive them. With VentiveIQ’s CPG data, you can personalize your approach towards these potential buyers, increasing the likelihood of a response and ultimately maximizing your campaign’s return on investment.
Conclusion
Overall, the use of data has become an essential component of modern marketing and business strategy. By leveraging data, brands can better understand their customers and make more informed decisions that drive success. And by using machine learning algorithms to analyze historical data and predict future trends, businesses can make more informed decisions, improve customer outcomes, and reduce costs. As predictive analytics continues to evolve, we can expect to see even more innovative ways in which businesses use data to drive success.