Data cleansing is the process of detecting and correcting incomplete data, essential for accurate audience segmentation, as it ensures that the data used to make decisions is reliable.
Introduction to Data
In today’s data-driven world, data cleansing has become an essential process for businesses to ensure that the data they use for analysis, decision-making, and strategy development is accurate, reliable, and consistent. Data cleansing, also known as data cleaning or data scrubbing, is the process of identifying and correcting or removing errors, inconsistencies, and inaccuracies in data.
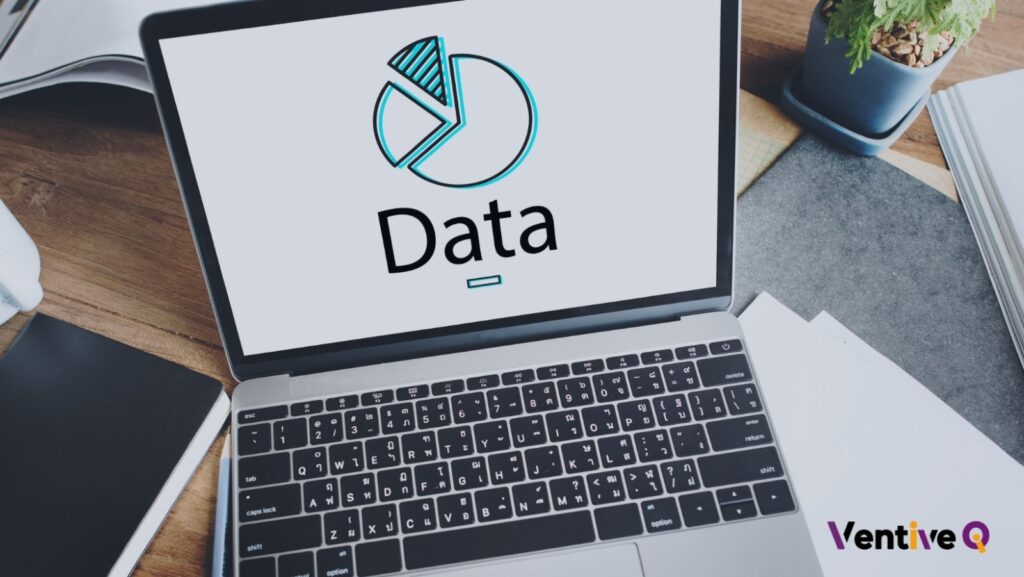
Data is an essential element of business operations. It is used to make strategic decisions, develop products and services, and enhance customer relationships. However, data is often cluttered and incomplete, making it challenging to extract valuable insights. This is where data cleansing becomes significant.
Know more about Identity Graph to unify your customer view.
What is Data Cleansing?
Data cleansing is the process of identifying and rectifying or removing errors, inconsistencies, and inaccuracies in data. It involves identifying incomplete, incorrect, or irrelevant information, and then replacing, modifying, or deleting it to ensure that the data is accurate, reliable, and consistent. It is also known as data cleaning or data scrubbing.
Data cleansing is a critical component of data quality management, which involves ensuring that data is accurate, complete, consistent, and timely. The process of data cleansing can be time-consuming and complex, but businesses need to ensure that they are using high-quality data for their operations.
Why Data Cleansing is Important?
Data cleansing is necessary for several reasons that you can read out below:
- Inaccurate data can lead to poor decision-making. For instance, if a company uses inaccurate sales data to forecast demand, it may order too much inventory, leading to excess costs and wasted resources. Contrarily, if the company uses insufficient data to forecast demand, it may not order enough inventory, leading to stockouts and missed sales opportunities.
- Data cleansing is important for compliance purposes. Many enterprises, such as healthcare and finance, are subject to regulatory requirements that mandate accurate and complete data. Not adhering to these requirements could lead to financial penalties, legal actions, and harm to the business’s reputation.
- Data cleansing is critical for maintaining customer trust. Inaccurate data can lead to incorrect billing, incorrect shipments, and other errors that can damage the company’s reputation and erode customer loyalty.
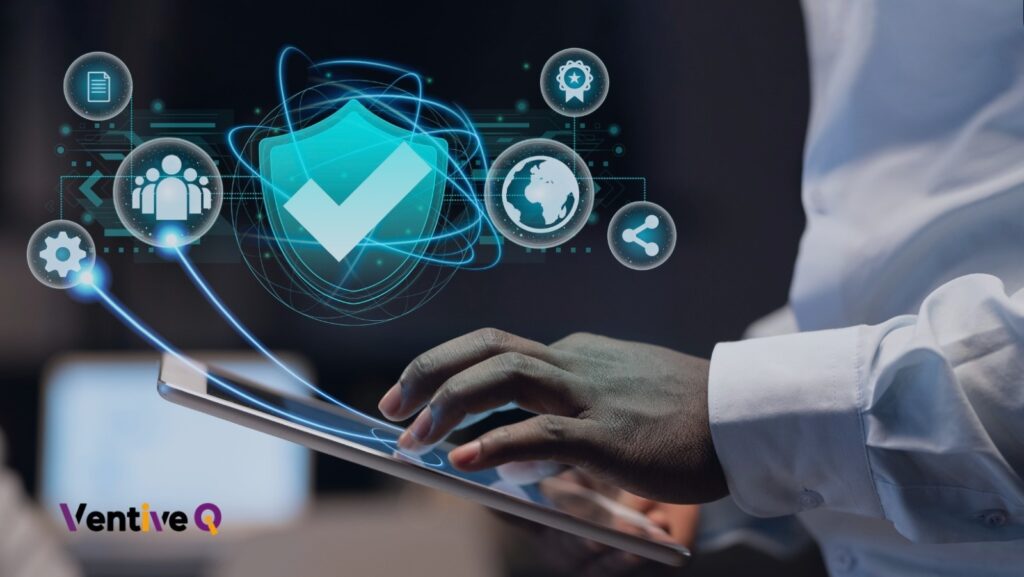
What are the Data Cleansing Techniques?
The process of data cleansing typically involves several steps. These steps may vary depending on the specific requirements of the business and the data being cleaned, but generally include the following:
See more: How Brands Can Leverage CTV and CPG Data for Better Marketing
- Data Profiling: Data profiling is the process of analyzing the data to identify any errors, inconsistencies, or inaccuracies. This can involve analyzing the data structure, identifying missing data, and examining data distributions to identify outliers and anomalies.
- Data Standardization: The process of data standardization ensures that data is maintained in a uniform format. This can involve standardizing dates, times, and units of measure, as well as converting text to a consistent case (uppercase or lowercase).
- Data Enrichment: Data enrichment involves adding further information to the data. This can include demographic or geographic data, such as zip codes or age ranges, as well as information about the products or services purchased.
- Data Validation: Data validation involves reviewing the data against external sources, such as reference data or industry standards, to ensure its accuracy and completeness. This can involve comparing the data to trusted sources, such as government databases, or using automated tools to detect anomalies.
- Data Deduplication: Data deduplication involves identifying and removing duplicate records from the data. This can involve comparing records based on certain fields, like name and address, and merging duplicate records into a single, proper record.
- Data Normalization: Data normalization involves ensuring that the data is in a consistent format. This can involve converting all text to uppercase or lowercase, as well as standardizing field names and formats.
What are the challenges in Data Cleansing?
Data cleansing can be a challenging process for businesses. Some of the key challenges that organizations face when cleansing their data include:
Read to know about the future of email marketing!
- Data Quality Issues: Data quality issues can occur due to various reasons, such as errors in data entry or processing, incomplete data, or missing data. These issues can make it difficult to clean the data and can require a considerable amount of time and resources to correct.
- Data Complexity: Data can be complex and tricky to understand, especially when dealing with large datasets or unstructured data such as text. This can make it challenging to pinpoint errors or inconsistencies in the data.
- Data Governance: Data governance refers to the processes, policies, and standards that organizations use to manage their data. A lack of effective data governance can make it tough to ensure that data is accurate, reliable, and consistent, and this can result in data quality issues.
- Data Privacy: Data privacy regulations, such as the GDPR and CCPA, require businesses to ensure that personal data is accurate and up to date. This can make it challenging to cleanse data that contains personal information, as businesses must ensure that they are complying with these regulations.
- Resource Constraints: Data cleansing can be a time-consuming and resource-intensive process. Businesses may not have the necessary resources or expertise to undertake data cleansing effectively, particularly if they have large datasets or complex data structures.
- Data Integration: Data cleansing can be challenging when integrating data from multiple sources, such as various systems or databases. Inconsistencies in data structures or formats can make it difficult to merge data effectively, which can result in errors or inconsistencies in the cleansed data.
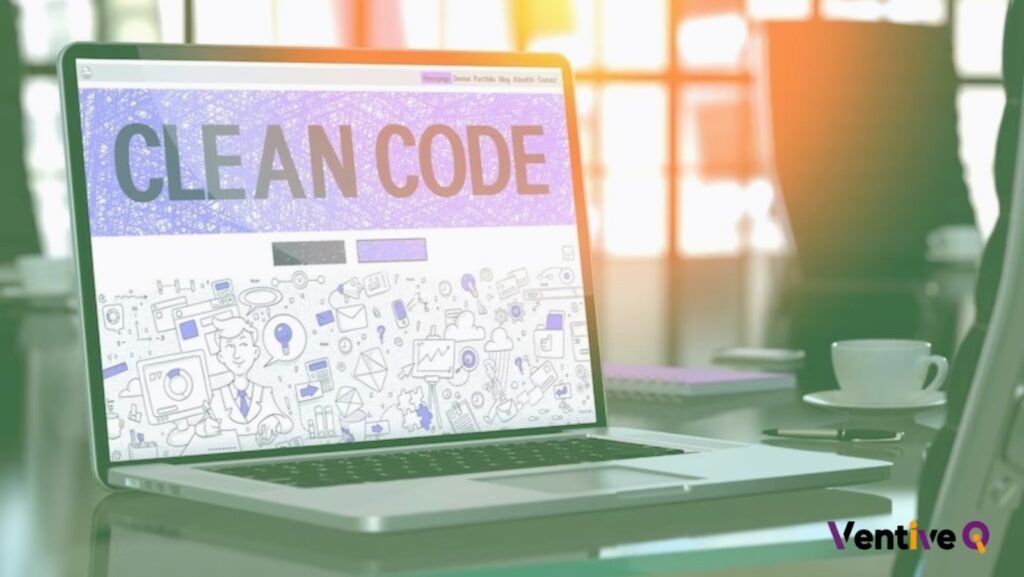
What are the Benefits of Data Cleansing?
Data cleansing offers several benefits to organizations, including:
1. Better Decision-Making
- Clean data enables organizations to make better decisions by providing accurate, reliable insights. When data is inaccurate, decision-makers may base their decisions on incorrect assumptions, leading to poor outcomes.
- However, when data is cleansed and validated, decision-makers can rely on the data to make informed decisions that lead to better outcomes.
2. Improved Customer Relationships
- Clean data can help organizations improve customer relationships by providing accurate billing, shipping, and other information.
- When customers receive incorrect information, it can damage their trust in the organization and lead to negative experiences.
- However, when organizations use clean data to communicate with customers, it can increase their satisfaction and loyalty.
3. Compliance with Regulations
- Many industries are subject to regulatory requirements that mandate accurate and complete data.
- By cleansing their data, organizations can ensure compliance with these regulations, reducing the risk of fines, legal action, and other penalties.
4. Increased Efficiency
- Data cleansing can help organizations increase efficiency by reducing the time and resources required to process data.
- When data is chaotic and incomplete, it takes longer to analyze and interpret, requiring more time and resources from data analysts and other personnel.
- However, when data is cleansed and standardized, it can be processed more quickly and efficiently, reducing the workload and freeing up resources for other tasks.
5. Improved Data Quality
- By cleansing their data, organizations can improve the overall quality of their data. This can lead to more accurate analyses and insights, as well as improved decision-making.
- Clean data also enables organizations to easily identify trends and patterns in the data, leading to greater innovation and competitive advantage.
What is Audience segmentation and its importance?
Audience segmentation is the process of dividing a target audience into smaller groups based on specific characteristics, such as demographics, behavior, interests, and preferences. Data cleansing is important for audience segmentation because it ensures that the data used to segment the audience is accurate and reliable.
If the data used to segment an audience is incomplete, incorrect, or inconsistent, it can lead to inaccurate and ineffective segmentation, which can result in a waste of resources and missed opportunities. For example, if a company wants to target customers based on their location, but the data they have is inaccurate, they may end up targeting the wrong people or missing out on potential customers.
Data cleansing is important for audience segmentation because it helps to ensure that the data used for segmentation is accurate and reliable, which in turn can lead to more effective targeting and better business results.
VentiveIQ role
VentiveIQ is a marketing agency that focuses on database marketing and excels in developing accurate targeting strategies across both traditional and digital channels. Among its clientele are some of the world’s leading organizations, including the largest healthcare insurance provider globally, the biggest telecommunication company in the US, and a few of the most esteemed universities. The agency assists its clients in identifying the ideal customers to expand their businesses.
Conclusion
Data cleansing is an essential process for organizations that rely on data to make strategic decisions and improve business outcomes. By identifying and correcting errors, inconsistencies, and inaccuracies in their data, organizations can ensure that their data is accurate, reliable, and consistent, leading to better decision-making, improved customer relationships, compliance with regulations, increased efficiency, and improved data quality.